A Journey towards Explainable AI and its Societal Implications
23rd November, 2020
Debasis Ganguly is a research staff member of IBM Research Europe, Dublin, Ireland. His research interests broadly span across topics such as semantic matching, trusted and fair AI, model interpretability, and AI for healthcare. He obtained his masters in computer science from the Indian Statistical Institute, Calcutta and his PhD on the topic of Information Retrieval from Dublin City University. He maintains an active academic profile by publishing in top-tier conferences and also acting as a PC member for conferences such as SIGIR, CIKM, WWW, NAACL, AAAI etc. He also regularly serves as a part of the reviewing committee for a number of high impact journals such as Pattern Recognition, IPM, TOIS etc. As workshop and shared task organizational experience, he has co-organized the two versions of the ‘Exploitation of Social Media for Emergency Relief and Preparedness (SMERP)’ workshops in ECIR 2017 and WWW 2018, and has co-organized the Personalized IR tracks at FIRE’11 and CLEF’17. He is currently organizing two shared tasks at FIRE-2020 on retrieval from conversational dialogues (RCD) and CAIR (Causality-driven Adhoc Information Retrieval).
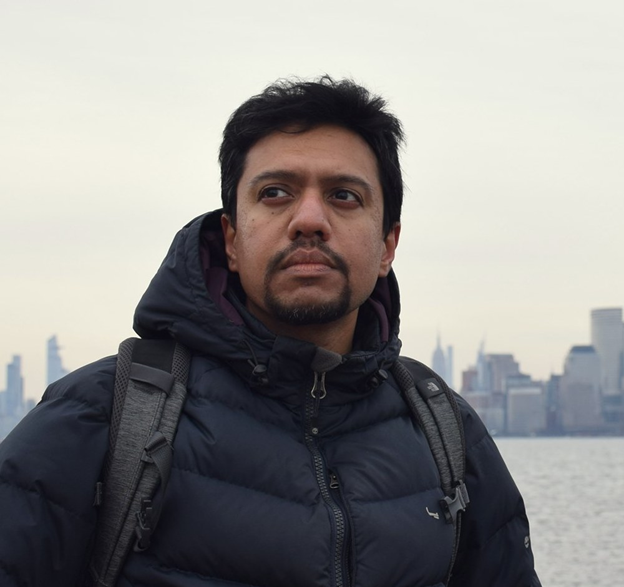
Dr. Debasis Ganguly
A research staff member of IBM Research Europe, Dublin, Ireland